Abstract
When we started working with artificial intelligence (AI) more than a decade ago, people were skeptical about whether this technology would develop enough in the foreseeable future to do anything useful. But we held on to our faith in AI’s potential to benefit humanity. We used games like chess, Go and Atari to train and test our AI systems to become smarter and more capable. In 2016, we decided to use our smart systems to try to solve a 50-year-old fundamental problem in biology, called the protein-folding problem. This was the birth of AlphaFold, our AI system that predicts the three-dimensional structures of proteins based on their amino acid sequence. In this article, you will learn about AlphaFold’s achievements, which demonstrate the power of AI to dramatically accelerate scientific discovery and benefit society.
Drs. Demis Hassabis and John Jumper won the 2023 Canada Gairdner International Award for developing AlphaFold, which is considered to be an AI-based solution to the 50-year grand challenge of predicting the structures of proteins based on their amino acid sequence. AlphaFold has been used to create the most accurate and complete picture of the human proteome—the set of all the proteins in the human body—with enormous potential to accelerate biological and medical research.
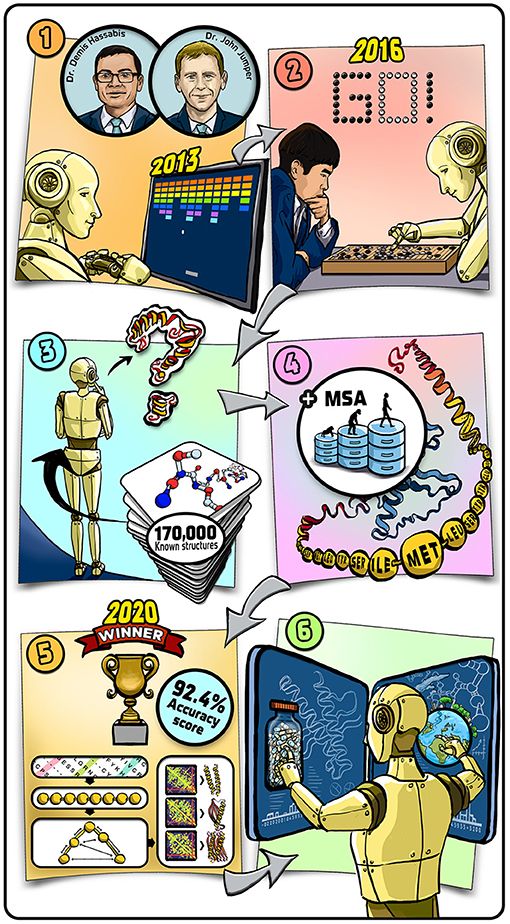
- Graphical Abstract - (1) We started our journey in 2013 by training our AI systems to play and win classic computer games.
- (2) We then moved to playing more complicated games against real people and, in 2016, our system won a challenge match of Go against the reigning world champion. (3) Shortly after, we began to tackle the protein-folding problem and trained our system on known protein structures. (4) To further train our system, we taught it to use additional databases containing information about how proteins evolved between species. (5) In 2020, our system achieved 92.4% average accuracy in the prediction of the three-dimensional structures of proteins. (6) We hope that our system will contribute to the development of new drugs, new tools for addressing climate change, and help scientists understand these tiny molecular machines that are the building blocks of life.
The Tiny Machines Of Life
Did you know that almost all the processes happening in your body are performed by tiny biological machines called proteins? Proteins help us to see, to move, to digest food, to fight diseases, and to perform many other essential actions needed to keep organisms like us alive and healthy (to learn more about proteins, check out this video). There are currently more than 200 million proteins known to science, and new proteins are discovered all the time.
Proteins are made of small building blocks called amino acids (to learn more about proteins and their composition, see this video). You can think of a protein like a string of beads, where the amino acids are the beads. There are 20 different amino acids, and they can be arranged in various combinations to make up a protein string. Proteins are made in a “factory” inside cells called the ribosome (to learn more about the ribosome, read this Nobel Collection article). In the ribosome, instructions from our genetic code (our DNA) get translated into chains of amino acids. Then, something amazing happens—these strings of amino acids fold up into complex, three-dimensional structures that in turn determine the functions proteins can perform.
A 50-Year-Old Problem
Since the early 1960s, scientists have been trying to understand exactly how the particular sequence of an amino acid chain results in the particular three-dimensional structure of a protein. This is known as the protein-folding problem [1]. Because proteins are so important for living things, the protein-folding problem was considered one of the most important problems in biochemistry. When scientists study any protein, they can easily determine which amino acids that protein contains—and even the exact order of amino acids in the protein string. But it has been much more difficult over the years to figure out the final three-dimensional shape that the string of amino acids folds into, to create the working protein machine. After all, proteins are much too small to simply examine under the microscope to see their shapes.
To figure out the three-dimensional structure of proteins, scientists have traditionally used a technique called X-ray crystallography (Figure 1). This involves crystallizing the protein, which means “freezing” many copies of it in a repeating 3D pattern. The crystallized protein is then examined using a huge machine that bounces high-energy X-rays off the protein (Figure 1A). Finally, the researcher must look at the patterns produced by those X-rays and perform very complex math to interpret the results and determine the actual structure of the protein. This process can take up to a few years for each protein! In the past 50 years, the structures of about 200,000 proteins have been determined by methods like X-ray crystallography, cryo-electron microscopy (to read more about cryo-electron microscopy, see here), and nuclear magnetic resonance analysis, and those structures have been made openly available in the Protein Data Bank.
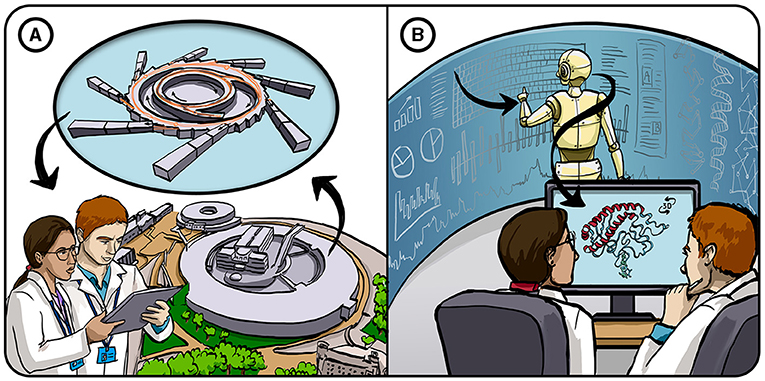
- Figure 1 - Tackling the protein-folding problem.
- (A) Traditionally, the structure of proteins has been determined by experiments that use very large, expensive machines to bounce X-rays off a crystallized protein (X-ray crystallography), followed by complex math to interpret the results. (B) Our approach at Google DeepMind is to use sophisticated AI systems that can use known protein structures and protein databases to learn to predict the structures of proteins that have not been experimentally tested yet. This approach saves a great deal of time and resources.
While this process has been successful, it is clearly too slow and expensive, especially if we want to find all the structures of the more than 200 million proteins that we know of. This is over 1,000 times more proteins than the number of structures we have determined so far!
Why is it so challenging to figure out the final three-dimensional shape of a protein? Well, just like a shoestring, there are an enormous number of ways that a chain of amino acids could potentially fold. Even a small protein, composed of just 150 amino acids, could be in as many as 10300 possible configurations (10300 is 1 followed by 300 zeroes—that is more than the number of stars in the universe!). With so many possible ways to fold a protein, how could scientists ever know which one is correct without doing time-consuming and expensive experiments like X-ray crystallography?
This is why, at Google DeepMind, we decided to use the power of artificial intelligence—the ability of computers to learn from examples and gain insights to solve complex problems—to tackle the protein-folding problem. This approach has proven very useful and saves a lot of time, money, and human effort while also giving us new insights into how proteins work (Figure 1B).
From Winning Games To Solving Scientific Problems
Our approach at Google DeepMind is to combine our passion for AI and our passion for science to find ways for AI to help humanity. At first, we taught our systems how to play simple computer games by teaching them the rules of the games and letting them improve through experience. Our next goal was to make these systems win more complex games, as a steppingstone to tackling difficult real-world problems. This included training an AI model to play a board game called Go, which is a very complex game with more than 10170 possible board configurations (more than the number of atoms in the known universe!). For a few years, we developed and tested AI systems in game situations, to see how well they were doing and to keep training them to get better. In 2016, one of our systems called AlphaGo defeated a world champion Go player named Lee Sedol—an achievement that was previously considered unimaginable. This was a huge steppingstone, and it proved that our AI systems were smart enough to deal with complex problems.
Google DeepMind has proud roots in scientific research, and so the protein-folding problem was a natural next step for us (Figure 2). Shortly after AlphaGo’s achievement in 2016, we assembled a team that started working on predicting the structures of proteins from their amino acid sequences. This new AI system was called AlphaFold (Figure 2A). AlphaFold was designed to learn from existing information about protein structures that had been published in open databases like the Protein Data Bank. Overall, we had access to about 170,000 known protein structures, which we used to train our AI system. We designed AlphaFold to process information somewhat similarly to the way the human brain does, using a computer science idea called artificial neural networks (to learn more about artificial neural networks and machine learning, read this Frontiers for Young Minds article). Like the human brain, AlphaFold can learn from experience and improve its performance. The more examples of protein structures we gave it, the better it got at predicting the structures of new proteins.
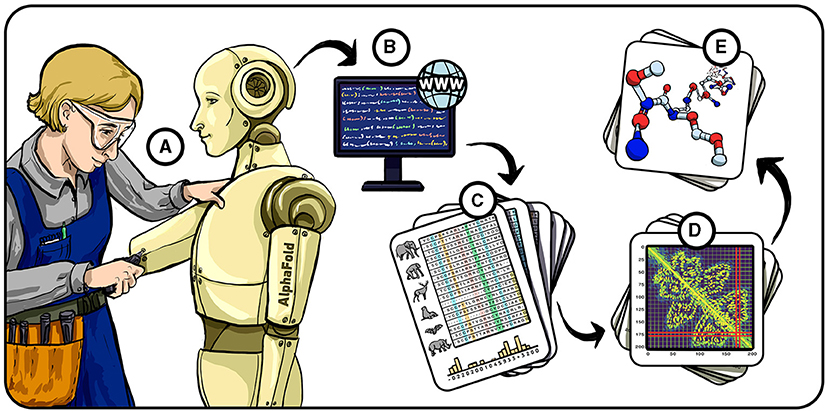
- Figure 2 - Stages in predicting protein folding.
- (A) In 2016, we started building AlphaFold—our AI system for tackling the protein-folding problem. (B) AlphaFold uses information from protein databases to train itself to predict a protein’s three-dimensional structure from its amino acid sequence. (C) We also trained AlphaFold using MSAs, which are groups of amino acid sequences from proteins that should have a similar structure, based on the functions they perform across multiple different organisms. The amino acids that change together, or “co-evolve,” between sequences (colorful columns) carry important information about which amino acids might be close together in the 3D structure. (D) Using the input information, AlphaFold predicts the distances and angles between every two amino acids. (E) Finally, AlphaFold translates the distances and angles into a predicted three-dimensional structure of the protein.
Unfortunately, even 170,000 examples were not enough to achieve the high level of performance we were looking for—we needed more information to train AlphaFold. So, we used open databases (Figure 2B) containing protein sequences to build what we call multiple sequence alignments (MSAs; Figure 2C). An MSA contains sequences that are evolutionarily related to the protein AlphaFold is making a prediction for, and together those sequences contain clues about the structure. The shapes of proteins determine the functions they can perform, and many organisms must perform the same biological function, such as carrying oxygen in the blood. This means that the three-dimensional structures of all oxygen-carrying proteins from different organisms probably stayed similar over the course of evolution, even if their underlying amino acid sequences changed. For that to happen, it means that whenever one amino acid changed in one place in the protein, another amino acid in the protein—the one closest to it in the three-dimensional structure—also had to change accordingly, to preserve the original shape. We call this co-evolution of amino acids, and by feeding this information into AlphaFold, we allowed the system to detect hidden relationships between amino acids.
Once we entered enough information into AlphaFold, the system could predict basic information about the shape of a protein, including the distances (Figure 2D) and angles between every two amino acids in the protein and the certainty of the prediction (how reliable it is). This information was “recycled” a few times within the system, and in each round AlphaFold improves its prediction. Finally it uses its basic idea of the protein shape to predict the 3D position of every atom in the protein structure (Figure 2E). When we started, we tested AlphaFold’s predictions on proteins whose structures were already known and let AlphaFold improve by learning from its errors and repeatedly correcting itself until its predictions became much better. After it was trained, we used the same network to run on unsolved structures and provide predictions for them.
The Evolution Of Alphafold
One exciting milestone in our journey with AlphaFold occurred in 2018, when AlphaFold came first in a biannual protein structure prediction challenge called CASP. AlphaFold received an average accuracy score of around 60 out of 100 on the hardest proteins [2], which was a great leap from the previous best score (which was about 40). This made us even more confident in AlphaFold’s capabilities, and we decided to improve the system even further for the next assessment. In our next version, called AlphaFold 2, we incorporated more of our scientific knowledge about the physics and geometry of amino acid chains into the system’s learning process and aligned it with everything we understood about the protein-folding problem. Essentially, we taught AlphaFold 2 how to perform MSA analysis, and then used that improved MSA analysis to gain a better understanding of protein folding (and therefore the physics and geometry of amino acid chains). This back-and-forth flow of information improved AlphaFold 2’s performance.
In the 2020 CASP14 structure prediction challenge, AlphaFold 2 won with an astounding accuracy score of 92.4 out of 100 [3]. This is approaching the accuracy of determining protein structures using experiments such as X-ray crystallography, but without the high time commitment or cost. Consequently, AlphaFold 2 was recognized as a solution to the 50-year-old protein-folding problem (see the CASP14 press release).
Even though this was a great achievement, it was still only the beginning. In 2020, we released the predicted protein structures of about 330,000 proteins and, by 2022, we did so for more than 200 million proteins. With time, the knowledge that we gain from all these structures will allow us to better understand protein biology and how proteins work together in cells. This capability will help so many people, from assisting in the development of new drugs and vaccines, to addressing climate change by designing new plastic-eating enzymes [4, 5]. AI systems like AlphaFold 2 could also speed up scientific discovery in general. Imagine how fast science could progress if we harnessed the great learning power of AI systems to tackle difficult problems in all fields of science and engineering. These are very exciting times, and we encourage you to stay informed and come along with us on this journey of using AI to unravel the most interesting mysteries of our world!
Glossary
Proteins: ↑ Tiny biological machines that perform most of the actions in our bodies.
Amino Acids: ↑ The building blocks of proteins.
Protein-Folding Problem: ↑ A scientific question posed in the 1960s asking how proteins fold to their three-dimensional structure based on their amino acid sequence.
X-ray Crystallography: ↑ An experimental method for determining the three-dimensional structure of protein using X-rays.
Artificial Intelligence: ↑ The ability of computers to learn like the human brain does and mimic human intelligence.
Multiple Sequence Alignments (MSAs): ↑ Amino acid sequences from proteins, found in different organisms, that should have similar structures based on their similar function.
Conflict of Interest
DH and JJ were employed by Google DeepMind.
Additional Materials
- 2023 Canada Gairdner International Award laureates: Dr. Demis Hassabis Dr. John Jumper.
- Drs. Demis Hassabis and John Jumper–2023 Canada Gairdner International Award (YouTube).
- Has Protein Folding Been Solved?—Sabine Hossenfelder (YouTube).
- DeepMind—Homepage.
Acknowledgments
We wish to thank Noa Segev for conducting the interview which served as the basis for this paper and for co-authoring the paper, and Iris Gat for providing the figures.
References
[1] ↑ Dill, K. A., Ozkan, S. B., Shell, M. S., and Weikl, T. R. 2008. The protein folding problem. Annu. Rev. Biophys. 37:289–316. doi: 10.1146/annurev.biophys.37.092707.153558
[2] ↑ Senior, A. W., Evans, R., Jumper, J., Kirkpatrick, J., Sifre, L., Green, T., et al. 2019. Protein structure prediction using multiple deep neural networks in the 13th Critical Assessment of Protein Structure Prediction (CASP13). Proteins 87:1141–8. doi: 10.1002/prot.25834
[3] ↑ Jumper, J., Evans, R., Pritzel, A., Green, T., Figurnov, M., Ronneberger, O., et al. 2021. Applying and improving AlphaFold at CASP14. Proteins 89:1711–21. doi: 10.1002/prot.26257
[4] ↑ Thornton, J. M., Laskowski, R. A., and Borkakoti, N. 2021. AlphaFold heralds a data-driven revolution in biology and medicine. Nat. Med. 27:1666–9. doi: 10.1038/s41591-021-01533-0
[5] ↑ Callaway, E. 2022. What’s next for the AI protein-folding revolution. Nature 604:234–8. doi: 10.1038/d41586-022-00997-5