Abstract
We receive bits of information every day. They come to us in a stream. When we listen to music, read a book, or solve a math problem we receive a stream of musical bits, word bits, or math bits. Our minds arrange that stream into a network. A network links together bits of information like musical notes, syllables, or math concepts. Networks help us to organize information and anticipate what is coming next. In this article, we ask two questions about how our minds build networks: First, are some networks easier to learn than others? And second, do we find some links between bits of information more surprising than others? The answer to both questions is “yes.” The findings reveal how humans learn about the networked world around them. Knowing how humans learn can also help us understand how to teach in ways that will result in the best learning.
What Is a Network?
Whether we realize it or not, our minds are constantly making predictions about what will happen next. If you see lightning, you might expect that you will hear thunder. If you see the letter A, you might expect that the letter B will follow. Learning the transitions between past events is important because our minds can use them to predict future events. The ability to make these predictions relies on networks, or webs of knowledge, built from observations and the connections between them [1].
Networks are made of nodes (things) and edges (connections between things) (Figure 1A). In the thunder and lightning example, the nodes are the thunder and lightning. They are connected by an edge, representing the connection or transition from one to the other. Networks are everywhere, connecting characters in a story, linking followers and followees on Instagram, and arranging syllables in language. To organize all this information, the human mind learns networks from past experiences1.
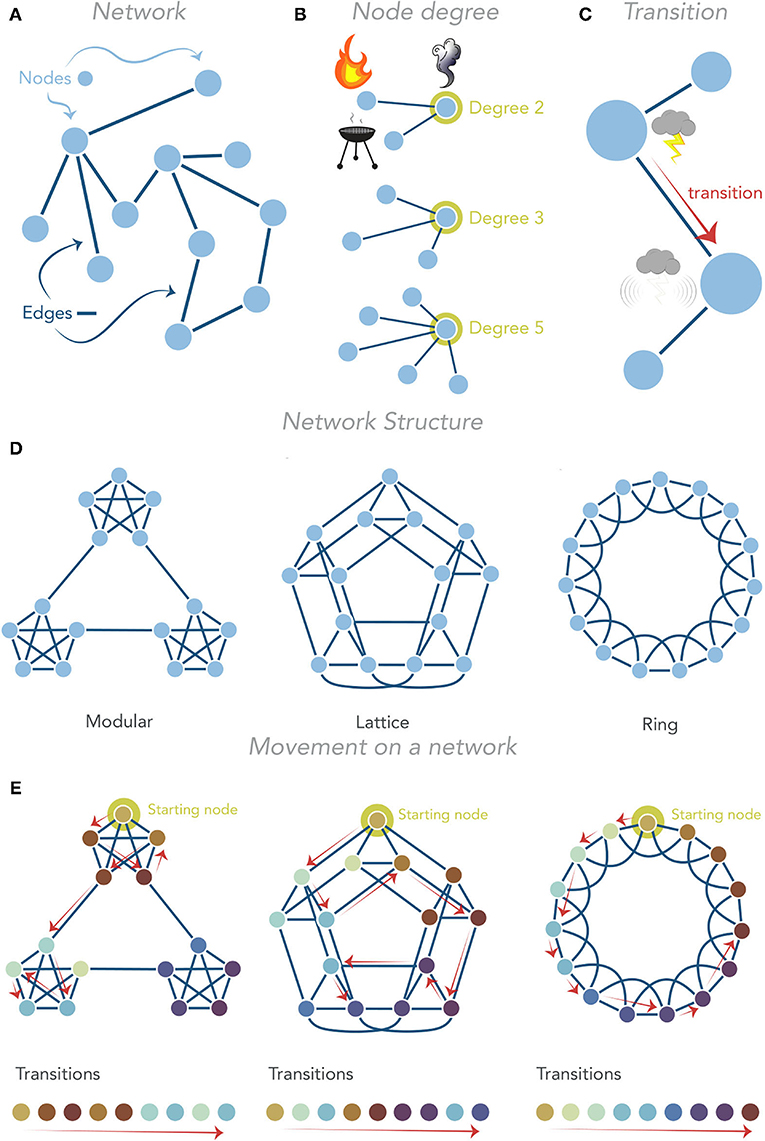
- Figure 1 - Properties of networks and network structures.
- (A) A network is a set of connected nodes. (B) The number of edges that a node has determines is its degree. (C) The edge between two nodes is called a transition. (D) The structure of networks can be modular, lattice, or ring. Modular networks have dense clusters. Lattice networks have evenly distributed edges. Edges in ring networks link neighboring nodes. (E) Transitions in modular networks mostly stay within clusters. Transitions in lattice networks move all over. Transitions in ring networks move to nearby nodes.
Why are networks so important? Networks help us to build a picture of the interconnected world. We can describe and measure networks by their properties. For example, the number of connections, or edges, extending from a node is called its degree (Figure 1B). When you smell smoke, you might see a fire or a barbeque. Therefore, the smoke node in this example has two edges, or a degree of two. Each edge, such as the link between lightning and thunder, represents a possible transition, or connection between the linked nodes (Figure 1C). Together, the connections between nodes define the network structure (or shape of the network). We will describe three types of network structures: a modular network, with three groups or clusters of nodes, a lattice network with repeated triangles, and a ring network in which nodes connect to other nodes close to them in a circle (Figure 1D). Think of each network structure as a different way you might learn information. If you want to learn about the history of America, you could ask your teacher, read a book, or use the internet. Each option could present the same information but might do so in a different order. This is just like how each network structure contains the same set of nodes, but a different set of transitions between them (Figure 1E).
Are Some Networks Easier to Learn Than Others?
Information is organized in a network. Think of a history lesson. Nodes represent certain objects, people, and events. Edges represent relationships between nodes. The lesson presents this information to us in an ordered sequence of nodes based on the edges. As we experience this sequence, our brains organize it into a mental network, linking the objects, people, and events that appear next to each other. In other words, the structures of our mental networks are based on the order of the information we receive. As we learn something really well, our mental networks come to resemble the original network of information that was presented.
Does a network’s shape make it easy or hard to learn? To find out, researchers compared modular and lattice networks. Both networks had the same 15 nodes, each connected to four other nodes (Figure 2) [2]. One group of participants learned a modular network and the other group learned a lattice network. Participants in this experiment sat in front of a computer screen and saw images that represented nodes. Regardless of the network they were learning, participants were shown the same 15 images. The only difference was the order of the sequence of images shown on the screen. Each image told the participants which buttons to press on a keyboard. It was just like pressing keys corresponding to notes in Guitar Hero or Piano Tiles. Importantly, an image could only follow another image in the sequence if the two images were connected in the network.
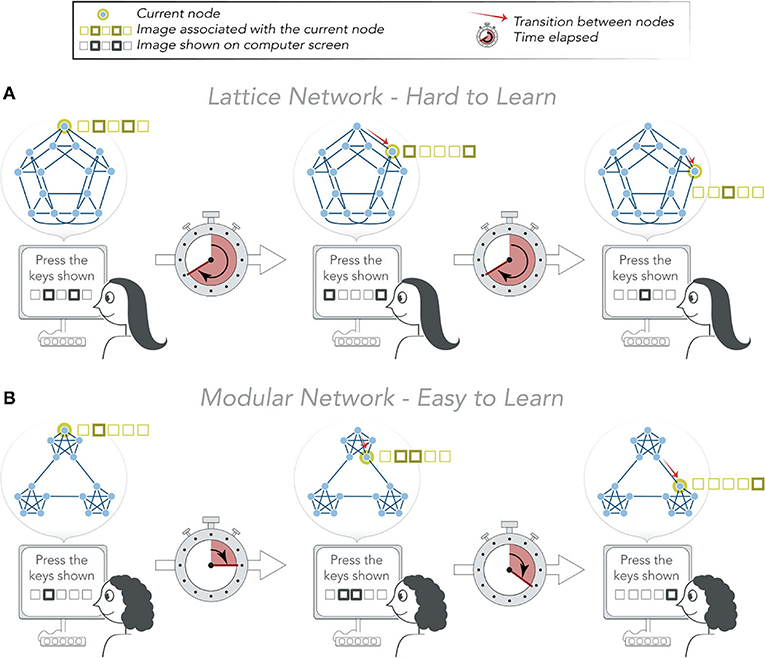
- Figure 2 - Are some networks easier to learn?
- Study participants were shown images on a computer screen that told them which buttons to press on a keyboard. Each image represented a node in the underlying network. After subjects pressed the correct keys, the screen would transition to a new image that was connected to the previous image in the network. (A) Participants responded slower to images arranged in a lattice structure. (B) People responded more quickly to images arranged in a modular structure, indicating that people learn more easily when information is organized in clusters.
Video 1 An animation of the experiment from the participant’s perspective. The video begins by introducing a network visually, highlighting what it looks like for two nodes to be connected vs. unconnected. Next, it illustrates the process of learning a network. A participant repeatedly experiences one node at a time on the screen and presses the corresponding buttons on a device, taking note of the transitions that occur. As time goes on, the participant’s learned network comes to match the computer’s hidden network. Finally, the video explains the significance of reaction times. Shorter reactions indicate that a network has been more thoroughly learned.
Participants never saw the network of connections between images (Figure 2). Instead, they only saw one image at a time. From the sequence of images, they had to learn the network of transitions. Think of a movie that you have watched so many times that you know exactly what each character will say next. Similarly, by observing transitions over and over, subjects slowly learned to predict the next image. To determine whether it was easier to learn using a modular network or a lattice network, researchers recorded how quickly participants reacted to each image by pressing the keys on the keyboard. A quick response indicated that the participant anticipated the transition. They had learned the network well. A slow response indicated that the transition surprised the subject. They had not learned the network well (Figure 2).
This experiment showed that the modular network was easier to learn than the lattice network. A modular network has multiple dense clusters of nodes, while a lattice network has no clusters. People could easily anticipate transitions in the modular network but not in the lattice network. This finding suggests that humans learn connections between people, objects, and events better when the information is presented in small tightly-connected groups. Second, the experiment showed that transitions within the same cluster are easier to learn than transitions between different clusters. Although modular networks are generally easier to learn, it is possible that some parts of a modular network are harder to learn than others. Overall, this experiment told scientists that clusters help humans learn networks of information and predict future events.
Are Some Connections More Surprising Than Others?
So, people can learn a network simply by observing transitions between nodes. But in real life, networks often change in unexpected ways. Our minds must face these changes and find new connections between previously unconnected ideas, events, and people2. What happens when new information does not fit into the network that we learned?
Once we learn a network, we come to expect transitions that we have seen before, and we are surprised by transitions that we have not seen before. A new transition “violates” the connections that we learned. Some new transitions surprise us more than others. To measure this experimentally, researchers used the ring network (Figure 3). Participants again observed sequences of images. After observing 500 transitions, occasionally, an image would appear that was not connected to the previous image in the network. This transition violated the rules of the network that the participants had learned. Due to the structure of the ring network, some violations were short: one image transitioned to another close by on the ring. Other violations were long: one image transitioned to another far away on the ring. The ring network shape made it easy for researchers to compare short and long violations.
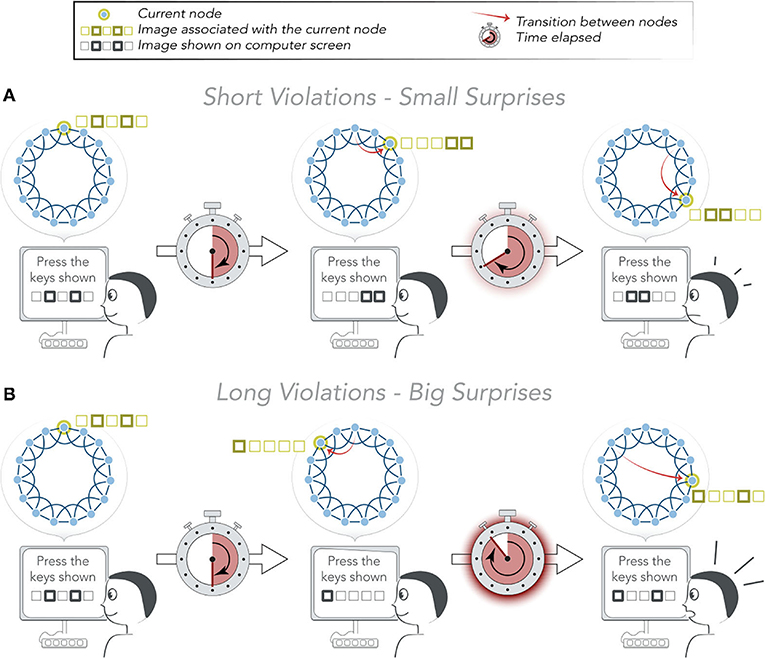
- Figure 3 - Humans are surprised by connections that violate the network that they learned.
- Subjects were shown images that told them which keys to press on a keyboard. These images transitioned from one to another according to an underlying network of connections between images, here a ring network. (A) When an image appeared that was not connected to the previous one (a network violation), subjects took longer to respond. (B) Subjects took even longer to respond to long violations that transitioned between images on opposite sides of the ring network than to short violations between images that were nearby on the ring.
Researchers could tell how surprising each violation was by measuring how long it took a participant to respond. You might expect participants to be equally surprised by all types of network violations. After all, each violation was totally new, having never appeared before. However, this was not the case. People were more surprised by long violations than by short violations. This finding indicates that people are less surprised when a new transition is close to a connection that already exists in the network. Think about each node as an event in your daily routine. Each morning you probably wake up, eat breakfast, brush your teeth, and then go to school. Imagine instead that you were told to brush your teeth before eating breakfast. You might feel slightly surprised. What if you were told to go to school before brushing your teeth? You would feel even more surprised. This finding suggests that our minds do not just learn the connections between things, they also learn the distances between things that are not directly connected.
Can We Design Networks That Are Easy to Learn?
We have just described two aspects of how people learn networks. First, people learn best when information is organized into clusters. Second, because surprise is an indication of how well something is learned, people are more surprised when they see something that is very different from what they have already learned. These discoveries are important because understanding how we learn can improve how we teach. Can teachers present information in a way that makes it easier to learn? Think of a history lesson. Usually, the information is presented in chronological order. However, we know that information is easier to learn when it is grouped into modules. What if history lessons were grouped by themes? Maybe all political events could be in one section. And maybe all science events could be in another section. Students would not have to remember facts that seem randomly connected. Instead, students would have a clear network of connections in which to place new information. This could help students learn more efficiently.
Choosing how to organize information does not only apply to the classroom. People of all ages are constantly reading, learning, and remembering [3]. Some people have jobs in which the information they learn has important effects on the world. For example, politicians often read science reports, which explain research findings and how they relate to policy decisions. The reports might explain how littering near a lake will harm local fish, for instance. How should scientists organize information to help politicians make the best decisions? By organizing information into modules, with clear connections within categories, scientists can communicate to non-scientists more effectively.
Glossary
Network: ↑ A set of nodes (things) connected by edges (relationships).
Node: ↑ A thing in a network that can be connected to other things.
Edge: ↑ A link that connects nodes in a network.
Degree: ↑ The number of other nodes to which a given node is connected.
Transition: ↑ The movement from one node to another connected node.
Network Structure: ↑ A network’s shape or what a network looks like, particularly its arrangement of nodes and edges.
Conflict of Interest
The authors declare that the research was conducted in the absence of any commercial or financial relationships that could be construed as a potential conflict of interest.
Original Source Article
↑Lynn, C. W., Kahn, A. E., Nyema, N., and Bassett, D. S. 2020. Abstract representations of events arise from mental errors in learning and memory. Nat. Commun. 11:2313. doi: 10.1038/s41467-020-15146-7
References
[1] ↑ Lynn, C. W., and Bassett, D. S. 2020. How humans learn and represent networks. Proc. Natl. Acad. Sci. U.S.A. 117:29407–15. doi: 10.1073/pnas.1912328117
[2] ↑ Lynn, C. W., Kahn, A. E., Nyema, N., and Bassett, D. S. 2020. Abstract representations of events arise from mental errors in learning and memory. Nat. Commun. 11:2313. doi: 10.1038/s41467-020-15146-7
[3] ↑ Bertolero, M. A., and Bassett, D. S. 2019. How the mind emerges from the brain’s complex networks. Sci. Am. 321:1. doi: 10.1038/scientificamerican0719-26
Footnotes
[1] ↑ https://penntoday.upenn.edu/news/err-human-learn-divine
[2] ↑ https://penntoday.upenn.edu/news/what-do-bohemian-rhapsody-macbeth-and-list-facebook-friends-all-have-common