Abstract
Health data—information from sources like medical records, surveys, and even electronic devices like smartwatches—are becoming increasingly important for keeping people healthy. Computers and the internet make it easy to store and share health data. Scientists and researchers can use these data to understand and prevent diseases or to develop better treatments. To do so, they combine biology, computer science, and math to understand data and find patterns. But using health data is not easy. Scientists must first find the right information among the many data sources available. They also need to make sure the data are “clean” and correct. Once health data are collected and checked, scientists analyze those data to make important discoveries. Health data are both personal and valuable, so they must be kept safe and private. By protecting people’s privacy, we encourage even more data sharing, which helps scientists learn even more and continue to improve human health.
Data = Health?
If you were to make a list of things that help you to stay healthy, what would be on it? You might include things like exercise, healthy food, plenty of sleep, and getting medical check-ups. But what about data, is that on your list? No? Well, maybe you will want to add it after reading this article! Health-related data—and lots of it—are becoming increasingly important for treating diseases and for keeping people from getting sick in the first place. The amount of health data being collected is growing exponentially. But what exactly is health data? And how can these data help keep people healthy? In this article, we will explain how some scientists are like data detectives, solving health mysteries with the help of data. If you are interested in how biology, medicine, and computer science can all work together to solve health puzzles, maybe you would like to be a data detective, too!
Data: Not Just for Laboratory Experiments Any More
“Data” used to be a term that was used mainly when talking about experiments done by laboratory scientists. Scientists would collect data from their experiments, analyze those data to see if they supported their hypotheses, and then use the data to plan their next experiments. Maybe a scientist’s data would eventually lead to a new medicine or a better understanding of a disease, but often the data (such as how many mice got better in response to a new drug, for example) were only used by the experimenters themselves.
The world of data, particularly health data, is changing. The term “health data” refers to any information related to people’s health. Health data can come from a variety of sources in addition to scientific research studies, including electronic medical records, patient surveys, DNA sequences, and data collected from a growing number of wearable devices, like smartwatches and blood sugar monitors. This is a HUGE amount of data, and thanks to computers and the internet, data are easier than ever to share. Data sharing allows many people—not just the scientists who created the data—to use data for things that can improve human health.
From “Big” Data to Better Health
The amount of health data being collected is so great that it can no longer simply be stored on a thumb drive—or even on a personal computer [1]. This incredible amount of data is often called “big data”, and it needs to be kept safe and organized. These huge sets of data are often stored on powerful computers called servers, which are meant to handle many tasks and “serve” many users at the same time. Servers are like huge digital libraries where scientists can keep and access their information (for more information on how servers work and to see what they look like, check out this video).
Health data on servers can also be shared with other researchers, who can act like detectives by analyzing the data to answer their own research questions—questions that the original data creators may never have even thought about! Using data in these new ways is called repurposing data, and it is very useful because researchers can come up with new discoveries without the need to do their own experiments from scratch. Repurposing data saves money and effort, and it can speed up the pace of scientific discoveries [2]. Discoveries made using health data can improve human health in many ways. For example, such discoveries could help prevent diseases from happening, detect and diagnose diseases that people already have, and treat diseases to help people get better—with fewer side effects (Figure 1).
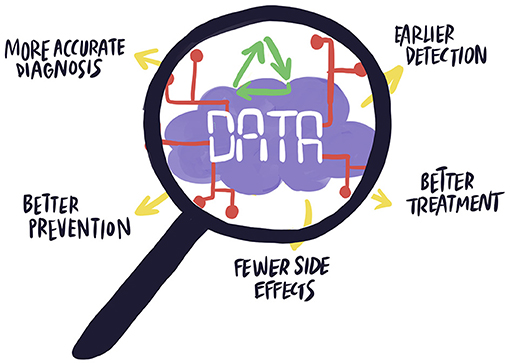
- Figure 1 - Huge amounts of health data are collected from many sources and stored on powerful computers called servers, which are like huge digital libraries.
- “Data detectives” who work in bioinformatics can access the servers and analyze these data to answer their own research questions, which may be quite different from the original purpose of the data. This is called data repurposing, and it can save money and time, speeding up scientific discoveries. Many important discoveries can be made from studying health data, including new ways to diagnose and detect diseases, better treatments for diseases, and even methods of preventing diseases altogether. Figure created by carlottacat.com.
However, getting from health data to actual improvements in people’s health is not an easy task—it requires a lot of detective work! An entire field of science, called bioinformatics, has developed to help with these discoveries. Bioinformatics combines aspects of biology, medicine, computer science, and mathematics to work with huge amounts of stored health data. In simple terms, bioinformatics is like a special kind of detective work that helps scientists sift through tons of data to solve mysteries about genes, proteins, and other important things that play a role in human health. Just as a detective uses clues to solve a case, bioinformaticians use computers and special software to find “clues” in “piles” of health data. But how do bioinformaticians help to make the leap from big data to better human health?
Data Discovery: Finding the Necessary Data
Imagine you were a data detective working in bioinformatics and you wanted to do a study on heart disease, for example. The first step is discovery—collecting all the data that are available on your topic. Like a detective working on a case, you must find the right data sources, depending on the type of data you need to solve your heart disease “puzzle”. Maybe some of the puzzle pieces in your heart disease mystery come from experiments and research that scientists have done in labs all around the world, in the form of DNA sequences or information about molecules related to heart disease. Other pieces of your puzzle might come from the electronic medical records of heart disease patients, or studies of how various drugs have affected such patients. Once you have found the right data sources, you must then make sure you can actually use those data. Some databases are open access, which means that anyone can use the data for free at any time and for any purpose, while other sources may be “closed”, meaning researchers must request access and meet certain requirements before they can use the data.
Say you have access to all your data sources. Remember that these sources contain data produced by other scientists or doctors, who might have been working on questions totally different from yours. That means there are probably all kinds of data you do not need mixed up with the data you do need—like pieces from a bunch of jigsaw puzzles, all mixed together. The challenge is finding your specific puzzle pieces amidst all the other data. Special bioinformatics tools are used to “ask questions” of the data, to pull out only the data that relate to your research question. For example, you could separate all the DNA sequences (or medical records) of people with heart disease from those of healthy people or people with other diseases.
Combining and Cleaning Data: Fitting the Pieces Together
As mentioned above, just like a detective must collect clues from multiple sources and suspects, chances are you will need more than one data source to solve your heart disease mystery. For instance, if you want to find out whether a mutation in a certain gene is linked to heart disease, you might need to combine electronic medical records (information about patients’ health histories and medical conditions) from one data source with DNA sequence data from a totally different database. The process of combining data from multiple sources is called data integration, and it helps bioinformaticians to spot relationships and patterns that they might not see using only one data source. Data integration can be complicated because data sources can use different formats, and they may not always be compatible with each other. Units of measurement might be different (pounds vs. kilograms or age vs. date of birth, for example) or the same kind of data might be called by different names in each source (last name vs. surname, for example). Data from separate sources must be made consistent before the sources can be combined.
To return to the puzzle analogy, imagine you were trying to assemble a puzzle, but you had extra copies of some of the pieces, and others were bent or broken so that they did not fit together well. You would probably want clean up the pieces as best you could, and remove the extra ones, to give yourself the best chance of successfully putting together the puzzle. In bioinformatics, this is where data cleaning comes in. Bioinformaticians must make sure, to the best of their ability, that all data are correct and ready to study. They use special computer programs and techniques to “clean up” the data and get rid of any mistakes or errors, like removing the same information that might have accidentally gotten into the data twice, or removing data points that are obviously wrong (for example, if a person’s age is listed as 273). Data cleaning is an especially important step, because errors in data can lead to incorrect conclusions—the way your puzzle might not look right if the pieces were messed up.
Data Analysis: Seeing the Whole Picture
Once your data are clean and ready, it is time for the fun part—data analysis. Bioinformaticians use powerful computers and clever mathematical procedures to do the “detective work” of looking for patterns and clues in data and drawing conclusions from their observations. This is the stage where you finally put your puzzle pieces together to reveal the overall picture—or the stage where the detective solves the mystery! In your study, you might discover that, of all people with heart disease, those with a certain gene are more likely to live longer if they take a certain drug, for instance. This type of conclusion would have been impossible to draw from looking at just medical records or just DNA sequences—but by using bioinformatics techniques to find, combine, clean, and analyze data, new relationships can be discovered that might eventually save patients’ lives (Figure 2).
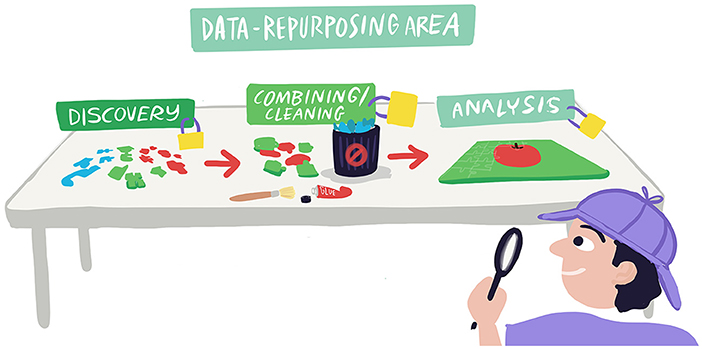
- Figure 2 - As “data detectives”, bioinformaticians must follow three basic steps when repurposing data.
- First, the right sources of data must be discovered. This is like gathering all the possible pieces to build a jigsaw puzzle. Data from various sources must be combined and cleaned, to make sure they are correct. This is like getting rid of any puzzle pieces that do not belong or fixing damaged pieces. Finally, data are analyzed to draw conclusions—like putting the puzzle together to see the whole picture! In each step of the process, there are important methods in place to keep personal health information safe and private. Figure created by carlottacat.com.
Keeping Data Safe and Private
Health data contain lots of personal information, so while this information is very important for the work of bioinformaticians and doctors, it must be kept safe and private. One way to keep data safe is to have special “locks” on the servers, to keep out everyone who does not have permission to see or use the information. Encryption is a process that can be used to protect health data, by turning the data into a kind of “secret code” that can only be decoded by people who have the “key” to the code (for more information on how encryption works, see this site). This way, even if a hacker or someone who is not authorized breaks the “locks” on a server and gets their hands on the encrypted data, they will not be able to understand it because it will look like gibberish. Sometimes, instead of (or along with) encryption, personal information such as name, address, or phone number can be removed from health data, so that no one will know who the remaining data belong to. Back-up copies of the data are also made, so that if something unexpected happens, like a computer problem or a serious natural disaster, the data will not be totally lost. For more information on heath data and issues of data privacy, see another article in this Collection.
While keeping health data safe and protected can be a big challenge, these steps are necessary to protect people’s privacy. When people trust that data-protection measures work and that their data are safe and anonymous, they may be more willing to share their data [3]. The more data sharing there is, the more information bioinformatics “data detectives” and other researchers have available to work with, as they continue to use data to solve mysteries that will improve human health!
Glossary
Health Data: ↑ Information about our bodies and how we take care of them. It helps scientists and doctors keep us healthy and find better treatments.
DNA Sequences: ↑ A special code in all cells that holds important information about our bodies. Scientists use DNA sequences to understand how our genes affect our health and to find ways to prevent or treat diseases.
Repurposing: ↑ Using existing data in new ways to make discoveries and improve our health without starting from scratch. It saves money and speeds up the pace of research.
Bioinformatics: ↑ A field that combines biology, computer science, and math. Scientists called bioinformaticians use computers to study and understand large amounts of data about our bodies and health.
Data Integration: ↑ The process of combining information from separate sources. Integration allows bioinformaticians to find connections and patterns that they would not see looking at just one source.
Data Cleaning: ↑ The process of making sure that the information in a dataset is accurate and reliable by fixing errors and removing duplicates, for example, to prepare data for analysis.
Data Analysis: ↑ Examining and studying data to find patterns, relationships, and important insights. Scientists use special tools and methods to make sense of the data and draw meaningful conclusions.
Encryption: ↑ A way of turning data into a secret code. Encryption keeps data safe and private because encrypted data can only be understood by those who have the special “key” to decode it.
Conflict of Interest
SD was employed by SJD Consulting LLC.
The remaining author declares that the research was conducted in the absence of any commercial or financial relationships that could be construed as a potential conflict of interest.
Acknowledgments
Articled inspired by the Sparks! Serendipity Forum at CERN. For more info on this particular topic, see talk by Rolf Apweiler.
References
[1] ↑ Culbertson, N. 2021. The Skyrocketing Volume of Healthcare Data Makes Privacy Imperative. Forbes Technology Council, Forbes (2021). Available online at: https://www.forbes.com/sites/forbestechcouncil/2021/08/06/the-skyrocketing-volume-of-healthcare-data-makes-privacy-imperative/?sh=3a724a606555 (accessed January 16, 2024).
[2] ↑ Sielemann, K., Hafner, A., and Pucker, B. 2020. The reuse of public datasets in the life sciences: potential risks and rewards. PeerJ Life Environ. 8:e9954. doi: 10.7717/peerj.9954
[3] ↑ Gille, F., Smith, S., and Mays, N. 2022. Evidence-based guiding principles to build public trust in personal data use in health systems. Digit. Health 8:20552076221111947. doi: 10.1177/20552076221111947